Nu kijken Deze tutorial heeft een gerelateerde videocursus gemaakt door het Real Python-team. Bekijk het samen met de geschreven tutorial om je begrip te verdiepen:Bestanden lezen en schrijven met Panda's
Panda's is een krachtig en flexibel Python-pakket waarmee u kunt werken met gelabelde en tijdreeksgegevens. Het biedt ook statistische methoden, maakt plotten mogelijk en meer. Een cruciaal kenmerk van Pandas is de mogelijkheid om Excel, CSV en vele andere soorten bestanden te schrijven en te lezen. Functies zoals de Panda's read_csv()
methode stelt u in staat effectief met bestanden te werken. U kunt ze gebruiken om de gegevens en labels van Pandas-objecten in een bestand op te slaan en ze later te laden als Pandas Series
of DataFrame
gevallen.
In deze tutorial leer je:
- Wat de Pandas IO-tools API is
- Hoe gegevens lezen en schrijven van en naar bestanden
- Hoe te werken met verschillende bestandsindelingen
- Hoe te werken met big data efficiënt
Laten we beginnen met het lezen en schrijven van bestanden!
Gratis bonus: 5 Thoughts On Python Mastery, een gratis cursus voor Python-ontwikkelaars die je de routekaart en de mindset laat zien die je nodig hebt om je Python-vaardigheden naar een hoger niveau te tillen.
Panda's installeren
De code in deze tutorial wordt uitgevoerd met CPython 3.7.4 en Pandas 0.25.1. Het zou handig zijn om ervoor te zorgen dat u de nieuwste versies van Python en Panda's op uw computer hebt staan. Misschien wil je een nieuwe virtuele omgeving maken en de afhankelijkheden voor deze tutorial installeren.
Ten eerste heb je de Pandas-bibliotheek nodig. Misschien heb je het al geïnstalleerd. Als je dat niet doet, kun je het installeren met pip:
$ pip install pandas
Zodra het installatieproces is voltooid, zou Pandas moeten zijn geïnstalleerd en gereed zijn.
Anaconda is een uitstekende Python-distributie die wordt geleverd met Python, veel handige pakketten zoals Panda's en een pakket- en omgevingsmanager genaamd Conda. Ga voor meer informatie over Anaconda naar Python instellen voor machine learning op Windows.
Als u geen Panda's in uw virtuele omgeving heeft, kunt u deze installeren met Conda:
$ conda install pandas
Conda is krachtig omdat het de afhankelijkheden en hun versies beheert. Voor meer informatie over het werken met Conda, kun je de officiële documentatie raadplegen.
Gegevens voorbereiden
In deze zelfstudie gebruik je de gegevens met betrekking tot 20 landen. Hier is een overzicht van de gegevens en bronnen waarmee u gaat werken:
-
Land wordt aangegeven met de naam van het land. Elk land staat in de top 10 van bevolking, oppervlakte of bruto binnenlands product (BBP). De rijlabels voor de dataset zijn de drieletterige landcodes die zijn gedefinieerd in ISO 3166-1. Het kolomlabel voor de dataset is
COUNTRY
. -
Bevolking wordt uitgedrukt in miljoenen. De gegevens komen uit een lijst van landen en afhankelijkheden per bevolking op Wikipedia. Het kolomlabel voor de dataset is
POP
. -
Gebied uitgedrukt in duizenden kilometers in het kwadraat. De gegevens komen uit een lijst van landen en afhankelijkheden per gebied op Wikipedia. Het kolomlabel voor de dataset is
AREA
. -
Bruto binnenlands product wordt uitgedrukt in miljoenen Amerikaanse dollars, volgens de gegevens van de Verenigde Naties voor 2017. U vindt deze gegevens in de lijst van landen naar nominaal BBP op Wikipedia. Het kolomlabel voor de dataset is
GDP
. -
Continent is ofwel Afrika, Azië, Oceanië, Europa, Noord-Amerika of Zuid-Amerika. Deze informatie vind je ook op Wikipedia. Het kolomlabel voor de dataset is
CONT
. -
Onafhankelijkheidsdag is een datum die de onafhankelijkheid van een land herdenkt. De gegevens komen uit de lijst met nationale onafhankelijkheidsdagen op Wikipedia. De datums worden weergegeven in ISO 8601-formaat. De eerste vier cijfers staan voor het jaar, de volgende twee cijfers voor de maand en de laatste twee voor de dag van de maand. Het kolomlabel voor de dataset is
IND_DAY
.
Zo zien de gegevens eruit als een tabel:
LAND | POP | GEBIED | BBP | CONT | IND_DAY | |
---|---|---|---|---|---|---|
CHN | China | 1398.72 | 9596,96 | 12234.78 | Azië | |
IND | India | 1351.16 | 3287.26 | 2575,67 | Azië | 1947-08-15 |
VS | VS | 329,74 | 9833.52 | 485,39 | N.Amerika | 1776-07-04 |
IDN | Indonesië | 268.07 | 1910.93 | 1015.54 | Azië | 1945-08-17 |
BH | Brazilië | 210.32 | 8515.77 | 2055.51 | Zuid-Amerika | 1822-09-07 |
PAK | Pakistan | 205,71 | 881.91 | 302.14 | Azië | 1947-08-14 |
NGA | Nigeria | 200,96 | 923.77 | 375,77 | Afrika | 1960-10-01 |
BGD | Bangladesh | 167.09 | 147.57 | 245.63 | Azië | 1971-03-26 |
RUS | Rusland | 146,79 | 17098.25 | 1530.75 | 1992-06-12 | |
MEX | Mexico | 126,58 | 1964.38 | 1158.23 | N.Amerika | 1810-09-16 |
JPN | Japan | 126.22 | 377,97 | 4872.42 | Azië | |
DEU | Duitsland | 83.02 | 357.11 | 3693.20 | Europa | |
FRA | Frankrijk | 67.02 | 640.68 | 2582.49 | Europa | 1789-07-14 |
GBR | VK | 66,44 | 242,50 | 2631.23 | Europa | |
ITA | Italië | 60.36 | 301.34 | 1943,84 | Europa | |
ARG | Argentinië | 44,94 | 2780.40 | 637.49 | Zuid-Amerika | 1816-07-09 |
DZA | Algerije | 43,38 | 2381.74 | 167.56 | Afrika | 1962-07-05 |
KAN | Canada | 37,59 | 9984.67 | 1647.12 | N.Amerika | 1867-07-01 |
AUS | Australië | 25.47 | 7692.02 | 1408.68 | Oceanië | |
KAZ | Kazachstan | 18.53 | 2724,90 | 159.41 | Azië | 1991/12/16 |
Het is u wellicht opgevallen dat sommige gegevens ontbreken. Het continent voor Rusland is bijvoorbeeld niet gespecificeerd omdat het zich over zowel Europa als Azië verspreidt. Er zijn ook verschillende ontbrekende onafhankelijkheidsdagen omdat de gegevensbron ze weglaat.
U kunt deze gegevens in Python ordenen met behulp van een genest woordenboek:
data = {
'CHN': {'COUNTRY': 'China', 'POP': 1_398.72, 'AREA': 9_596.96,
'GDP': 12_234.78, 'CONT': 'Asia'},
'IND': {'COUNTRY': 'India', 'POP': 1_351.16, 'AREA': 3_287.26,
'GDP': 2_575.67, 'CONT': 'Asia', 'IND_DAY': '1947-08-15'},
'USA': {'COUNTRY': 'US', 'POP': 329.74, 'AREA': 9_833.52,
'GDP': 19_485.39, 'CONT': 'N.America',
'IND_DAY': '1776-07-04'},
'IDN': {'COUNTRY': 'Indonesia', 'POP': 268.07, 'AREA': 1_910.93,
'GDP': 1_015.54, 'CONT': 'Asia', 'IND_DAY': '1945-08-17'},
'BRA': {'COUNTRY': 'Brazil', 'POP': 210.32, 'AREA': 8_515.77,
'GDP': 2_055.51, 'CONT': 'S.America', 'IND_DAY': '1822-09-07'},
'PAK': {'COUNTRY': 'Pakistan', 'POP': 205.71, 'AREA': 881.91,
'GDP': 302.14, 'CONT': 'Asia', 'IND_DAY': '1947-08-14'},
'NGA': {'COUNTRY': 'Nigeria', 'POP': 200.96, 'AREA': 923.77,
'GDP': 375.77, 'CONT': 'Africa', 'IND_DAY': '1960-10-01'},
'BGD': {'COUNTRY': 'Bangladesh', 'POP': 167.09, 'AREA': 147.57,
'GDP': 245.63, 'CONT': 'Asia', 'IND_DAY': '1971-03-26'},
'RUS': {'COUNTRY': 'Russia', 'POP': 146.79, 'AREA': 17_098.25,
'GDP': 1_530.75, 'IND_DAY': '1992-06-12'},
'MEX': {'COUNTRY': 'Mexico', 'POP': 126.58, 'AREA': 1_964.38,
'GDP': 1_158.23, 'CONT': 'N.America', 'IND_DAY': '1810-09-16'},
'JPN': {'COUNTRY': 'Japan', 'POP': 126.22, 'AREA': 377.97,
'GDP': 4_872.42, 'CONT': 'Asia'},
'DEU': {'COUNTRY': 'Germany', 'POP': 83.02, 'AREA': 357.11,
'GDP': 3_693.20, 'CONT': 'Europe'},
'FRA': {'COUNTRY': 'France', 'POP': 67.02, 'AREA': 640.68,
'GDP': 2_582.49, 'CONT': 'Europe', 'IND_DAY': '1789-07-14'},
'GBR': {'COUNTRY': 'UK', 'POP': 66.44, 'AREA': 242.50,
'GDP': 2_631.23, 'CONT': 'Europe'},
'ITA': {'COUNTRY': 'Italy', 'POP': 60.36, 'AREA': 301.34,
'GDP': 1_943.84, 'CONT': 'Europe'},
'ARG': {'COUNTRY': 'Argentina', 'POP': 44.94, 'AREA': 2_780.40,
'GDP': 637.49, 'CONT': 'S.America', 'IND_DAY': '1816-07-09'},
'DZA': {'COUNTRY': 'Algeria', 'POP': 43.38, 'AREA': 2_381.74,
'GDP': 167.56, 'CONT': 'Africa', 'IND_DAY': '1962-07-05'},
'CAN': {'COUNTRY': 'Canada', 'POP': 37.59, 'AREA': 9_984.67,
'GDP': 1_647.12, 'CONT': 'N.America', 'IND_DAY': '1867-07-01'},
'AUS': {'COUNTRY': 'Australia', 'POP': 25.47, 'AREA': 7_692.02,
'GDP': 1_408.68, 'CONT': 'Oceania'},
'KAZ': {'COUNTRY': 'Kazakhstan', 'POP': 18.53, 'AREA': 2_724.90,
'GDP': 159.41, 'CONT': 'Asia', 'IND_DAY': '1991-12-16'}
}
columns = ('COUNTRY', 'POP', 'AREA', 'GDP', 'CONT', 'IND_DAY')
Elke rij van de tabel is geschreven als een inner dictionary waarvan de sleutels de kolomnamen zijn en de waarden de corresponderende gegevens. Deze woordenboeken worden vervolgens verzameld als de waarden in de buitenste data
woordenboek. De bijbehorende sleutels voor data
zijn de drieletterige landcodes.
U kunt deze data
gebruiken om een instantie van een Pandas DataFrame
te maken . Eerst moet je Panda's importeren:
>>> import pandas as pd
Nu je Panda's hebt geïmporteerd, kun je het DataFrame
. gebruiken constructor en data
om een DataFrame
te maken voorwerp.
data
is zo georganiseerd dat de landcodes overeenkomen met kolommen. U kunt de rijen en kolommen van een DataFrame
omdraaien met de eigenschap .T
:
>>> df = pd.DataFrame(data=data).T
>>> df
COUNTRY POP AREA GDP CONT IND_DAY
CHN China 1398.72 9596.96 12234.8 Asia NaN
IND India 1351.16 3287.26 2575.67 Asia 1947-08-15
USA US 329.74 9833.52 19485.4 N.America 1776-07-04
IDN Indonesia 268.07 1910.93 1015.54 Asia 1945-08-17
BRA Brazil 210.32 8515.77 2055.51 S.America 1822-09-07
PAK Pakistan 205.71 881.91 302.14 Asia 1947-08-14
NGA Nigeria 200.96 923.77 375.77 Africa 1960-10-01
BGD Bangladesh 167.09 147.57 245.63 Asia 1971-03-26
RUS Russia 146.79 17098.2 1530.75 NaN 1992-06-12
MEX Mexico 126.58 1964.38 1158.23 N.America 1810-09-16
JPN Japan 126.22 377.97 4872.42 Asia NaN
DEU Germany 83.02 357.11 3693.2 Europe NaN
FRA France 67.02 640.68 2582.49 Europe 1789-07-14
GBR UK 66.44 242.5 2631.23 Europe NaN
ITA Italy 60.36 301.34 1943.84 Europe NaN
ARG Argentina 44.94 2780.4 637.49 S.America 1816-07-09
DZA Algeria 43.38 2381.74 167.56 Africa 1962-07-05
CAN Canada 37.59 9984.67 1647.12 N.America 1867-07-01
AUS Australia 25.47 7692.02 1408.68 Oceania NaN
KAZ Kazakhstan 18.53 2724.9 159.41 Asia 1991-12-16
Nu heb je je DataFrame
object gevuld met de gegevens over elk land.
Opmerking: U kunt .transpose()
. gebruiken in plaats van .T
om de rijen en kolommen van uw dataset om te draaien. Als u .transpose()
. gebruikt , dan kunt u de optionele parameter copy
. instellen om aan te geven of u de onderliggende gegevens wilt kopiëren. Het standaardgedrag is False
.
Versies van Python ouder dan 3.6 gaven geen garantie voor de volgorde van sleutels in woordenboeken. Om ervoor te zorgen dat de volgorde van kolommen behouden blijft voor oudere versies van Python en Panda's, kunt u index=columns
opgeven :
>>> df = pd.DataFrame(data=data, index=columns).T
Nu je je gegevens hebt voorbereid, ben je klaar om met bestanden te gaan werken!
De Panda's gebruiken read_csv()
en .to_csv()
Functies
Een bestand met door komma's gescheiden waarden (CSV) is een bestand met platte tekst met een .csv
extensie die tabelgegevens bevat. Dit is een van de meest populaire bestandsindelingen voor het opslaan van grote hoeveelheden gegevens. Elke rij van het CSV-bestand vertegenwoordigt een enkele tabelrij. De waarden in dezelfde rij worden standaard gescheiden door komma's, maar u kunt het scheidingsteken wijzigen in een puntkomma, tab, spatie of een ander teken.
Schrijf een CSV-bestand
U kunt uw Panda's DataFrame
. opslaan als een CSV-bestand met .to_csv()
:
>>> df.to_csv('data.csv')
Dat is het! U heeft het bestand data.csv
gemaakt in uw huidige werkmap. U kunt het onderstaande codeblok uitvouwen om te zien hoe uw CSV-bestand eruit moet zien:
,COUNTRY,POP,AREA,GDP,CONT,IND_DAY
CHN,China,1398.72,9596.96,12234.78,Asia,
IND,India,1351.16,3287.26,2575.67,Asia,1947-08-15
USA,US,329.74,9833.52,19485.39,N.America,1776-07-04
IDN,Indonesia,268.07,1910.93,1015.54,Asia,1945-08-17
BRA,Brazil,210.32,8515.77,2055.51,S.America,1822-09-07
PAK,Pakistan,205.71,881.91,302.14,Asia,1947-08-14
NGA,Nigeria,200.96,923.77,375.77,Africa,1960-10-01
BGD,Bangladesh,167.09,147.57,245.63,Asia,1971-03-26
RUS,Russia,146.79,17098.25,1530.75,,1992-06-12
MEX,Mexico,126.58,1964.38,1158.23,N.America,1810-09-16
JPN,Japan,126.22,377.97,4872.42,Asia,
DEU,Germany,83.02,357.11,3693.2,Europe,
FRA,France,67.02,640.68,2582.49,Europe,1789-07-14
GBR,UK,66.44,242.5,2631.23,Europe,
ITA,Italy,60.36,301.34,1943.84,Europe,
ARG,Argentina,44.94,2780.4,637.49,S.America,1816-07-09
DZA,Algeria,43.38,2381.74,167.56,Africa,1962-07-05
CAN,Canada,37.59,9984.67,1647.12,N.America,1867-07-01
AUS,Australia,25.47,7692.02,1408.68,Oceania,
KAZ,Kazakhstan,18.53,2724.9,159.41,Asia,1991-12-16
Dit tekstbestand bevat de gegevens gescheiden door komma's . De eerste kolom bevat de rijlabels. In sommige gevallen vindt u ze niet relevant. Als u ze niet wilt behouden, kunt u het argument index=False
. doorgeven naar .to_csv()
.
Lees een CSV-bestand
Zodra uw gegevens zijn opgeslagen in een CSV-bestand, wilt u deze waarschijnlijk van tijd tot tijd laden en gebruiken. Dat kan met de Panda's read_csv()
functie:
>>> df = pd.read_csv('data.csv', index_col=0)
>>> df
COUNTRY POP AREA GDP CONT IND_DAY
CHN China 1398.72 9596.96 12234.78 Asia NaN
IND India 1351.16 3287.26 2575.67 Asia 1947-08-15
USA US 329.74 9833.52 19485.39 N.America 1776-07-04
IDN Indonesia 268.07 1910.93 1015.54 Asia 1945-08-17
BRA Brazil 210.32 8515.77 2055.51 S.America 1822-09-07
PAK Pakistan 205.71 881.91 302.14 Asia 1947-08-14
NGA Nigeria 200.96 923.77 375.77 Africa 1960-10-01
BGD Bangladesh 167.09 147.57 245.63 Asia 1971-03-26
RUS Russia 146.79 17098.25 1530.75 NaN 1992-06-12
MEX Mexico 126.58 1964.38 1158.23 N.America 1810-09-16
JPN Japan 126.22 377.97 4872.42 Asia NaN
DEU Germany 83.02 357.11 3693.20 Europe NaN
FRA France 67.02 640.68 2582.49 Europe 1789-07-14
GBR UK 66.44 242.50 2631.23 Europe NaN
ITA Italy 60.36 301.34 1943.84 Europe NaN
ARG Argentina 44.94 2780.40 637.49 S.America 1816-07-09
DZA Algeria 43.38 2381.74 167.56 Africa 1962-07-05
CAN Canada 37.59 9984.67 1647.12 N.America 1867-07-01
AUS Australia 25.47 7692.02 1408.68 Oceania NaN
KAZ Kazakhstan 18.53 2724.90 159.41 Asia 1991-12-16
In dit geval, de Panda's read_csv()
functie retourneert een nieuw DataFrame
met de gegevens en labels uit het bestand data.csv
, die u hebt opgegeven met het eerste argument. Deze tekenreeks kan elk geldig pad zijn, inclusief URL's.
De parameter index_col
specificeert de kolom uit het CSV-bestand die de rijlabels bevat. U wijst een op nul gebaseerde kolomindex toe aan deze parameter. U moet de waarde van index_col
. bepalen wanneer het CSV-bestand de rijlabels bevat om te voorkomen dat ze als gegevens worden geladen.
Later in deze zelfstudie leert u meer over het gebruik van Panda's met CSV-bestanden. Je kunt ook lezen en schrijven van CSV-bestanden in Python om te zien hoe je met CSV-bestanden omgaat met de ingebouwde Python-bibliotheek csv.
Panda's gebruiken om Excel-bestanden te schrijven en te lezen
Microsoft Excel is waarschijnlijk de meest gebruikte spreadsheetsoftware. Terwijl oudere versies binaire .xls
gebruikten bestanden, introduceerde Excel 2007 de nieuwe op XML gebaseerde .xlsx
het dossier. U kunt Excel-bestanden lezen en schrijven in Panda's, vergelijkbaar met CSV-bestanden. U moet echter eerst de volgende Python-pakketten installeren:
- xlwt om naar
.xls
te schrijven bestanden - openpyxl of XlsxWriter om naar
.xlsx
te schrijven bestanden - xlrd om Excel-bestanden te lezen
Je kunt ze installeren met pip met een enkele opdracht:
$ pip install xlwt openpyxl xlsxwriter xlrd
Je kunt Conda ook gebruiken:
$ conda install xlwt openpyxl xlsxwriter xlrd
Houd er rekening mee dat u niet alles hoeft te installeren deze pakketten. U hebt bijvoorbeeld niet zowel openpyxl als XlsxWriter nodig. Als je alleen met .xls
gaat werken bestanden, dan heb je ze niet nodig! Als u echter alleen met .xlsx
wilt werken, bestanden, dan heb je er minstens één nodig, maar niet xlwt
. Neem even de tijd om te beslissen welke pakketten geschikt zijn voor uw project.
Schrijf een Excel-bestand
Zodra u deze pakketten hebt geïnstalleerd, kunt u uw DataFrame
. opslaan in een Excel-bestand met .to_excel()
:
>>> df.to_excel('data.xlsx')
Het argument 'data.xlsx'
vertegenwoordigt het doelbestand en, optioneel, het pad. De bovenstaande verklaring zou het bestand data.xlsx
moeten maken in uw huidige werkmap. Dat bestand zou er als volgt uit moeten zien:
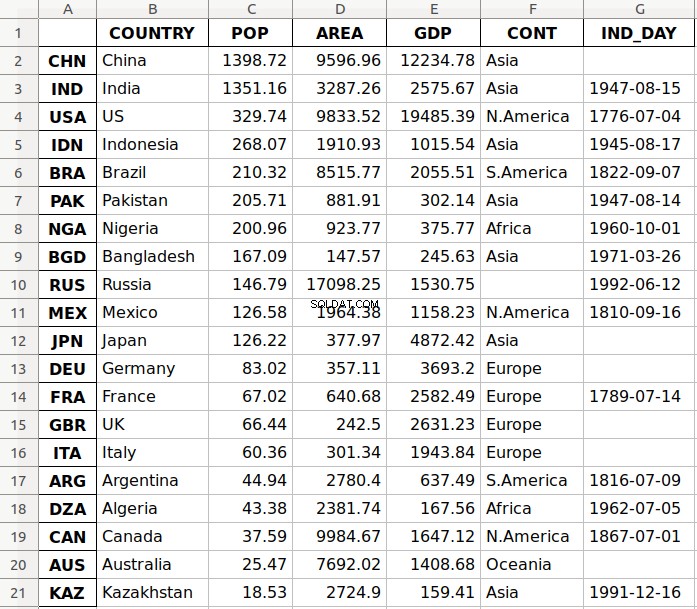
De eerste kolom van het bestand bevat de labels van de rijen, terwijl de andere kolommen gegevens opslaan.
Een Excel-bestand lezen
U kunt gegevens uit Excel-bestanden laden met read_excel()
:
>>> df = pd.read_excel('data.xlsx', index_col=0)
>>> df
COUNTRY POP AREA GDP CONT IND_DAY
CHN China 1398.72 9596.96 12234.78 Asia NaN
IND India 1351.16 3287.26 2575.67 Asia 1947-08-15
USA US 329.74 9833.52 19485.39 N.America 1776-07-04
IDN Indonesia 268.07 1910.93 1015.54 Asia 1945-08-17
BRA Brazil 210.32 8515.77 2055.51 S.America 1822-09-07
PAK Pakistan 205.71 881.91 302.14 Asia 1947-08-14
NGA Nigeria 200.96 923.77 375.77 Africa 1960-10-01
BGD Bangladesh 167.09 147.57 245.63 Asia 1971-03-26
RUS Russia 146.79 17098.25 1530.75 NaN 1992-06-12
MEX Mexico 126.58 1964.38 1158.23 N.America 1810-09-16
JPN Japan 126.22 377.97 4872.42 Asia NaN
DEU Germany 83.02 357.11 3693.20 Europe NaN
FRA France 67.02 640.68 2582.49 Europe 1789-07-14
GBR UK 66.44 242.50 2631.23 Europe NaN
ITA Italy 60.36 301.34 1943.84 Europe NaN
ARG Argentina 44.94 2780.40 637.49 S.America 1816-07-09
DZA Algeria 43.38 2381.74 167.56 Africa 1962-07-05
CAN Canada 37.59 9984.67 1647.12 N.America 1867-07-01
AUS Australia 25.47 7692.02 1408.68 Oceania NaN
KAZ Kazakhstan 18.53 2724.90 159.41 Asia 1991-12-16
read_excel()
retourneert een nieuw DataFrame
die de waarden bevat van data.xlsx
. U kunt ook read_excel()
. gebruiken met OpenDocument-spreadsheets of .ods
bestanden.
Later in deze zelfstudie leert u meer over het werken met Excel-bestanden. Je kunt ook Panda's gebruiken om grote Excel-bestanden in Python te lezen bekijken.
De Pandas IO API begrijpen
Pandas IO-tools is de API waarmee u de inhoud van Series
. kunt opslaan en DataFrame
objecten naar het klembord, objecten of bestanden van verschillende typen. Het maakt het ook mogelijk om gegevens van het klembord, objecten of bestanden te laden.
Bestanden schrijven
Series
en DataFrame
objecten hebben methoden waarmee gegevens en labels naar het klembord of bestanden kunnen worden geschreven. Ze worden genoemd met het patroon .to_<file-type>()
, waar <file-type>
is het type van het doelbestand.
Je hebt geleerd over .to_csv()
en .to_excel()
, maar er zijn andere, waaronder:
.to_json()
.to_html()
.to_sql()
.to_pickle()
Er zijn nog meer bestandstypen waarnaar u kunt schrijven, dus deze lijst is niet uitputtend.
Opmerking: Raadpleeg de officiële documentatie over serialisatie, IO en conversie met betrekking tot Series
om vergelijkbare methoden te vinden. en DataFrame
objecten.
Deze methoden hebben parameters die het doelbestandspad specificeren waar u de gegevens en labels hebt opgeslagen. In sommige gevallen is dit verplicht en in andere gevallen optioneel. Als deze optie beschikbaar is en u ervoor kiest om deze weg te laten, retourneren de methoden de objecten (zoals strings of iterables) met de inhoud van DataFrame
gevallen.
De optionele parameter compression
bepaalt hoe het bestand wordt gecomprimeerd met de gegevens en labels. U leert er later meer over. Er zijn een paar andere parameters, maar deze zijn meestal specifiek voor een of meerdere methoden. U gaat hier niet in detail op in.
Bestanden lezen
Panda's-functies voor het lezen van de inhoud van bestanden worden benoemd met het patroon .read_<file-type>()
, waar <file-type>
geeft het type bestand aan dat moet worden gelezen. Je hebt de Panda's al gezien read_csv()
en read_excel()
functies. Hier zijn er nog een paar:
read_json()
read_html()
read_sql()
read_pickle()
Deze functies hebben een parameter die het doelbestandspad specificeert. Het kan elke geldige tekenreeks zijn die het pad vertegenwoordigt, op een lokale computer of in een URL. Andere objecten zijn ook acceptabel, afhankelijk van het bestandstype.
De optionele parameter compression
bepaalt het type decompressie dat moet worden gebruikt voor de gecomprimeerde bestanden. U zult er later in deze zelfstudie meer over leren. Er zijn andere parameters, maar deze zijn specifiek voor een of meerdere functies. U gaat hier niet in detail op in.
Werken met verschillende bestandstypen
De Pandas-bibliotheek biedt een breed scala aan mogelijkheden voor het opslaan van uw gegevens in bestanden en het laden van gegevens uit bestanden. In dit gedeelte leert u meer over het werken met CSV- en Excel-bestanden. Je zult ook zien hoe je andere soorten bestanden kunt gebruiken, zoals JSON, webpagina's, databases en Python-pickle-bestanden.
CSV-bestanden
U hebt al geleerd hoe u CSV-bestanden kunt lezen en schrijven. Laten we nu wat dieper ingaan op de details. Wanneer u .to_csv()
gebruikt om uw DataFrame
op te slaan , kunt u een argument opgeven voor de parameter path_or_buf
om het pad, de naam en de extensie van het doelbestand op te geven.
path_or_buf
is het eerste argument .to_csv()
zal krijgen. Het kan elke tekenreeks zijn die een geldig bestandspad vertegenwoordigt dat de bestandsnaam en de extensie bevat. Je hebt dit in een eerder voorbeeld gezien. Als u echter path_or_buf
. weglaat , dan .to_csv()
zal geen bestanden maken. In plaats daarvan zal het de corresponderende string teruggeven:
>>> df = pd.DataFrame(data=data).T
>>> s = df.to_csv()
>>> print(s)
,COUNTRY,POP,AREA,GDP,CONT,IND_DAY
CHN,China,1398.72,9596.96,12234.78,Asia,
IND,India,1351.16,3287.26,2575.67,Asia,1947-08-15
USA,US,329.74,9833.52,19485.39,N.America,1776-07-04
IDN,Indonesia,268.07,1910.93,1015.54,Asia,1945-08-17
BRA,Brazil,210.32,8515.77,2055.51,S.America,1822-09-07
PAK,Pakistan,205.71,881.91,302.14,Asia,1947-08-14
NGA,Nigeria,200.96,923.77,375.77,Africa,1960-10-01
BGD,Bangladesh,167.09,147.57,245.63,Asia,1971-03-26
RUS,Russia,146.79,17098.25,1530.75,,1992-06-12
MEX,Mexico,126.58,1964.38,1158.23,N.America,1810-09-16
JPN,Japan,126.22,377.97,4872.42,Asia,
DEU,Germany,83.02,357.11,3693.2,Europe,
FRA,France,67.02,640.68,2582.49,Europe,1789-07-14
GBR,UK,66.44,242.5,2631.23,Europe,
ITA,Italy,60.36,301.34,1943.84,Europe,
ARG,Argentina,44.94,2780.4,637.49,S.America,1816-07-09
DZA,Algeria,43.38,2381.74,167.56,Africa,1962-07-05
CAN,Canada,37.59,9984.67,1647.12,N.America,1867-07-01
AUS,Australia,25.47,7692.02,1408.68,Oceania,
KAZ,Kazakhstan,18.53,2724.9,159.41,Asia,1991-12-16
Nu heb je de string s
in plaats van een CSV-bestand. U heeft ook enkele ontbrekende waarden in uw DataFrame
voorwerp. Het continent voor Rusland en de onafhankelijkheidsdagen voor verschillende landen (China, Japan, enzovoort) zijn bijvoorbeeld niet beschikbaar. In datawetenschap en machine learning moet je zorgvuldig omgaan met ontbrekende waarden. Panda's blinken hier uit! Standaard gebruikt Pandas de NaN-waarde om de ontbrekende waarden te vervangen.
Opmerking: nan
, wat staat voor "geen getal", is een bepaalde drijvende-kommawaarde in Python.
Je kunt een nan
. krijgen waarde met een van de volgende functies:
float('nan')
math.nan
numpy.nan
Het continent dat overeenkomt met Rusland in df
is nan
:
>>> df.loc['RUS', 'CONT']
nan
Dit voorbeeld gebruikt .loc[]
om gegevens te krijgen met de opgegeven rij- en kolomnamen.
Wanneer u uw DataFrame
. opslaat naar een CSV-bestand, lege tekenreeksen (''
) zal de ontbrekende gegevens vertegenwoordigen. Je kunt dit zowel zien in je bestand data.csv
en in de string s
. Als je dit gedrag wilt veranderen, gebruik dan de optionele parameter na_rep
:
>>> df.to_csv('new-data.csv', na_rep='(missing)')
Deze code produceert het bestand new-data.csv
waarbij de ontbrekende waarden niet langer lege tekenreeksen zijn. U kunt het onderstaande codeblok uitvouwen om te zien hoe dit bestand eruit moet zien:
,COUNTRY,POP,AREA,GDP,CONT,IND_DAY
CHN,China,1398.72,9596.96,12234.78,Asia,(missing)
IND,India,1351.16,3287.26,2575.67,Asia,1947-08-15
USA,US,329.74,9833.52,19485.39,N.America,1776-07-04
IDN,Indonesia,268.07,1910.93,1015.54,Asia,1945-08-17
BRA,Brazil,210.32,8515.77,2055.51,S.America,1822-09-07
PAK,Pakistan,205.71,881.91,302.14,Asia,1947-08-14
NGA,Nigeria,200.96,923.77,375.77,Africa,1960-10-01
BGD,Bangladesh,167.09,147.57,245.63,Asia,1971-03-26
RUS,Russia,146.79,17098.25,1530.75,(missing),1992-06-12
MEX,Mexico,126.58,1964.38,1158.23,N.America,1810-09-16
JPN,Japan,126.22,377.97,4872.42,Asia,(missing)
DEU,Germany,83.02,357.11,3693.2,Europe,(missing)
FRA,France,67.02,640.68,2582.49,Europe,1789-07-14
GBR,UK,66.44,242.5,2631.23,Europe,(missing)
ITA,Italy,60.36,301.34,1943.84,Europe,(missing)
ARG,Argentina,44.94,2780.4,637.49,S.America,1816-07-09
DZA,Algeria,43.38,2381.74,167.56,Africa,1962-07-05
CAN,Canada,37.59,9984.67,1647.12,N.America,1867-07-01
AUS,Australia,25.47,7692.02,1408.68,Oceania,(missing)
KAZ,Kazakhstan,18.53,2724.9,159.41,Asia,1991-12-16
Now, the string '(missing)'
in the file corresponds to the nan
values from df
.
When Pandas reads files, it considers the empty string (''
) and a few others as missing values by default:
'nan'
'-nan'
'NA'
'N/A'
'NaN'
'null'
If you don’t want this behavior, then you can pass keep_default_na=False
to the Pandas read_csv()
function. To specify other labels for missing values, use the parameter na_values
:
>>> pd.read_csv('new-data.csv', index_col=0, na_values='(missing)')
COUNTRY POP AREA GDP CONT IND_DAY
CHN China 1398.72 9596.96 12234.78 Asia NaN
IND India 1351.16 3287.26 2575.67 Asia 1947-08-15
USA US 329.74 9833.52 19485.39 N.America 1776-07-04
IDN Indonesia 268.07 1910.93 1015.54 Asia 1945-08-17
BRA Brazil 210.32 8515.77 2055.51 S.America 1822-09-07
PAK Pakistan 205.71 881.91 302.14 Asia 1947-08-14
NGA Nigeria 200.96 923.77 375.77 Africa 1960-10-01
BGD Bangladesh 167.09 147.57 245.63 Asia 1971-03-26
RUS Russia 146.79 17098.25 1530.75 NaN 1992-06-12
MEX Mexico 126.58 1964.38 1158.23 N.America 1810-09-16
JPN Japan 126.22 377.97 4872.42 Asia NaN
DEU Germany 83.02 357.11 3693.20 Europe NaN
FRA France 67.02 640.68 2582.49 Europe 1789-07-14
GBR UK 66.44 242.50 2631.23 Europe NaN
ITA Italy 60.36 301.34 1943.84 Europe NaN
ARG Argentina 44.94 2780.40 637.49 S.America 1816-07-09
DZA Algeria 43.38 2381.74 167.56 Africa 1962-07-05
CAN Canada 37.59 9984.67 1647.12 N.America 1867-07-01
AUS Australia 25.47 7692.02 1408.68 Oceania NaN
KAZ Kazakhstan 18.53 2724.90 159.41 Asia 1991-12-16
Here, you’ve marked the string '(missing)'
as a new missing data label, and Pandas replaced it with nan
when it read the file.
When you load data from a file, Pandas assigns the data types to the values of each column by default. You can check these types with .dtypes
:
>>> df = pd.read_csv('data.csv', index_col=0)
>>> df.dtypes
COUNTRY object
POP float64
AREA float64
GDP float64
CONT object
IND_DAY object
dtype: object
The columns with strings and dates ('COUNTRY'
, 'CONT'
, and 'IND_DAY'
) have the data type object
. Meanwhile, the numeric columns contain 64-bit floating-point numbers (float64
).
You can use the parameter dtype
to specify the desired data types and parse_dates
to force use of datetimes:
>>> dtypes = {'POP': 'float32', 'AREA': 'float32', 'GDP': 'float32'}
>>> df = pd.read_csv('data.csv', index_col=0, dtype=dtypes,
... parse_dates=['IND_DAY'])
>>> df.dtypes
COUNTRY object
POP float32
AREA float32
GDP float32
CONT object
IND_DAY datetime64[ns]
dtype: object
>>> df['IND_DAY']
CHN NaT
IND 1947-08-15
USA 1776-07-04
IDN 1945-08-17
BRA 1822-09-07
PAK 1947-08-14
NGA 1960-10-01
BGD 1971-03-26
RUS 1992-06-12
MEX 1810-09-16
JPN NaT
DEU NaT
FRA 1789-07-14
GBR NaT
ITA NaT
ARG 1816-07-09
DZA 1962-07-05
CAN 1867-07-01
AUS NaT
KAZ 1991-12-16
Name: IND_DAY, dtype: datetime64[ns]
Now, you have 32-bit floating-point numbers (float32
) as specified with dtype
. These differ slightly from the original 64-bit numbers because of smaller precision . The values in the last column are considered as dates and have the data type datetime64
. That’s why the NaN
values in this column are replaced with NaT
.
Now that you have real dates, you can save them in the format you like:
>>>>>> df = pd.read_csv('data.csv', index_col=0, parse_dates=['IND_DAY'])
>>> df.to_csv('formatted-data.csv', date_format='%B %d, %Y')
Here, you’ve specified the parameter date_format
to be '%B %d, %Y'
. You can expand the code block below to see the resulting file:
,COUNTRY,POP,AREA,GDP,CONT,IND_DAY
CHN,China,1398.72,9596.96,12234.78,Asia,
IND,India,1351.16,3287.26,2575.67,Asia,"August 15, 1947"
USA,US,329.74,9833.52,19485.39,N.America,"July 04, 1776"
IDN,Indonesia,268.07,1910.93,1015.54,Asia,"August 17, 1945"
BRA,Brazil,210.32,8515.77,2055.51,S.America,"September 07, 1822"
PAK,Pakistan,205.71,881.91,302.14,Asia,"August 14, 1947"
NGA,Nigeria,200.96,923.77,375.77,Africa,"October 01, 1960"
BGD,Bangladesh,167.09,147.57,245.63,Asia,"March 26, 1971"
RUS,Russia,146.79,17098.25,1530.75,,"June 12, 1992"
MEX,Mexico,126.58,1964.38,1158.23,N.America,"September 16, 1810"
JPN,Japan,126.22,377.97,4872.42,Asia,
DEU,Germany,83.02,357.11,3693.2,Europe,
FRA,France,67.02,640.68,2582.49,Europe,"July 14, 1789"
GBR,UK,66.44,242.5,2631.23,Europe,
ITA,Italy,60.36,301.34,1943.84,Europe,
ARG,Argentina,44.94,2780.4,637.49,S.America,"July 09, 1816"
DZA,Algeria,43.38,2381.74,167.56,Africa,"July 05, 1962"
CAN,Canada,37.59,9984.67,1647.12,N.America,"July 01, 1867"
AUS,Australia,25.47,7692.02,1408.68,Oceania,
KAZ,Kazakhstan,18.53,2724.9,159.41,Asia,"December 16, 1991"
The format of the dates is different now. The format '%B %d, %Y'
means the date will first display the full name of the month, then the day followed by a comma, and finally the full year.
There are several other optional parameters that you can use with .to_csv()
:
sep
denotes a values separator.decimal
indicates a decimal separator.encoding
sets the file encoding.header
specifies whether you want to write column labels in the file.
Here’s how you would pass arguments for sep
and header
:
>>> s = df.to_csv(sep=';', header=False)
>>> print(s)
CHN;China;1398.72;9596.96;12234.78;Asia;
IND;India;1351.16;3287.26;2575.67;Asia;1947-08-15
USA;US;329.74;9833.52;19485.39;N.America;1776-07-04
IDN;Indonesia;268.07;1910.93;1015.54;Asia;1945-08-17
BRA;Brazil;210.32;8515.77;2055.51;S.America;1822-09-07
PAK;Pakistan;205.71;881.91;302.14;Asia;1947-08-14
NGA;Nigeria;200.96;923.77;375.77;Africa;1960-10-01
BGD;Bangladesh;167.09;147.57;245.63;Asia;1971-03-26
RUS;Russia;146.79;17098.25;1530.75;;1992-06-12
MEX;Mexico;126.58;1964.38;1158.23;N.America;1810-09-16
JPN;Japan;126.22;377.97;4872.42;Asia;
DEU;Germany;83.02;357.11;3693.2;Europe;
FRA;France;67.02;640.68;2582.49;Europe;1789-07-14
GBR;UK;66.44;242.5;2631.23;Europe;
ITA;Italy;60.36;301.34;1943.84;Europe;
ARG;Argentina;44.94;2780.4;637.49;S.America;1816-07-09
DZA;Algeria;43.38;2381.74;167.56;Africa;1962-07-05
CAN;Canada;37.59;9984.67;1647.12;N.America;1867-07-01
AUS;Australia;25.47;7692.02;1408.68;Oceania;
KAZ;Kazakhstan;18.53;2724.9;159.41;Asia;1991-12-16
The data is separated with a semicolon (';'
) because you’ve specified sep=';'
. Also, since you passed header=False
, you see your data without the header row of column names.
The Pandas read_csv()
function has many additional options for managing missing data, working with dates and times, quoting, encoding, handling errors, and more. For instance, if you have a file with one data column and want to get a Series
object instead of a DataFrame
, then you can pass squeeze=True
to read_csv()
. You’ll learn later on about data compression and decompression, as well as how to skip rows and columns.
JSON Files
JSON stands for JavaScript object notation. JSON files are plaintext files used for data interchange, and humans can read them easily. They follow the ISO/IEC 21778:2017 and ECMA-404 standards and use the .json
extension. Python and Pandas work well with JSON files, as Python’s json library offers built-in support for them.
You can save the data from your DataFrame
to a JSON file with .to_json()
. Start by creating a DataFrame
object again. Use the dictionary data
that holds the data about countries and then apply .to_json()
:
>>> df = pd.DataFrame(data=data).T
>>> df.to_json('data-columns.json')
This code produces the file data-columns.json
. You can expand the code block below to see how this file should look:
{"COUNTRY":{"CHN":"China","IND":"India","USA":"US","IDN":"Indonesia","BRA":"Brazil","PAK":"Pakistan","NGA":"Nigeria","BGD":"Bangladesh","RUS":"Russia","MEX":"Mexico","JPN":"Japan","DEU":"Germany","FRA":"France","GBR":"UK","ITA":"Italy","ARG":"Argentina","DZA":"Algeria","CAN":"Canada","AUS":"Australia","KAZ":"Kazakhstan"},"POP":{"CHN":1398.72,"IND":1351.16,"USA":329.74,"IDN":268.07,"BRA":210.32,"PAK":205.71,"NGA":200.96,"BGD":167.09,"RUS":146.79,"MEX":126.58,"JPN":126.22,"DEU":83.02,"FRA":67.02,"GBR":66.44,"ITA":60.36,"ARG":44.94,"DZA":43.38,"CAN":37.59,"AUS":25.47,"KAZ":18.53},"AREA":{"CHN":9596.96,"IND":3287.26,"USA":9833.52,"IDN":1910.93,"BRA":8515.77,"PAK":881.91,"NGA":923.77,"BGD":147.57,"RUS":17098.25,"MEX":1964.38,"JPN":377.97,"DEU":357.11,"FRA":640.68,"GBR":242.5,"ITA":301.34,"ARG":2780.4,"DZA":2381.74,"CAN":9984.67,"AUS":7692.02,"KAZ":2724.9},"GDP":{"CHN":12234.78,"IND":2575.67,"USA":19485.39,"IDN":1015.54,"BRA":2055.51,"PAK":302.14,"NGA":375.77,"BGD":245.63,"RUS":1530.75,"MEX":1158.23,"JPN":4872.42,"DEU":3693.2,"FRA":2582.49,"GBR":2631.23,"ITA":1943.84,"ARG":637.49,"DZA":167.56,"CAN":1647.12,"AUS":1408.68,"KAZ":159.41},"CONT":{"CHN":"Asia","IND":"Asia","USA":"N.America","IDN":"Asia","BRA":"S.America","PAK":"Asia","NGA":"Africa","BGD":"Asia","RUS":null,"MEX":"N.America","JPN":"Asia","DEU":"Europe","FRA":"Europe","GBR":"Europe","ITA":"Europe","ARG":"S.America","DZA":"Africa","CAN":"N.America","AUS":"Oceania","KAZ":"Asia"},"IND_DAY":{"CHN":null,"IND":"1947-08-15","USA":"1776-07-04","IDN":"1945-08-17","BRA":"1822-09-07","PAK":"1947-08-14","NGA":"1960-10-01","BGD":"1971-03-26","RUS":"1992-06-12","MEX":"1810-09-16","JPN":null,"DEU":null,"FRA":"1789-07-14","GBR":null,"ITA":null,"ARG":"1816-07-09","DZA":"1962-07-05","CAN":"1867-07-01","AUS":null,"KAZ":"1991-12-16"}}
data-columns.json
has one large dictionary with the column labels as keys and the corresponding inner dictionaries as values.
You can get a different file structure if you pass an argument for the optional parameter orient
:
>>> df.to_json('data-index.json', orient='index')
The orient
parameter defaults to 'columns'
. Here, you’ve set it to index
.
You should get a new file data-index.json
. You can expand the code block below to see the changes:
{"CHN":{"COUNTRY":"China","POP":1398.72,"AREA":9596.96,"GDP":12234.78,"CONT":"Asia","IND_DAY":null},"IND":{"COUNTRY":"India","POP":1351.16,"AREA":3287.26,"GDP":2575.67,"CONT":"Asia","IND_DAY":"1947-08-15"},"USA":{"COUNTRY":"US","POP":329.74,"AREA":9833.52,"GDP":19485.39,"CONT":"N.America","IND_DAY":"1776-07-04"},"IDN":{"COUNTRY":"Indonesia","POP":268.07,"AREA":1910.93,"GDP":1015.54,"CONT":"Asia","IND_DAY":"1945-08-17"},"BRA":{"COUNTRY":"Brazil","POP":210.32,"AREA":8515.77,"GDP":2055.51,"CONT":"S.America","IND_DAY":"1822-09-07"},"PAK":{"COUNTRY":"Pakistan","POP":205.71,"AREA":881.91,"GDP":302.14,"CONT":"Asia","IND_DAY":"1947-08-14"},"NGA":{"COUNTRY":"Nigeria","POP":200.96,"AREA":923.77,"GDP":375.77,"CONT":"Africa","IND_DAY":"1960-10-01"},"BGD":{"COUNTRY":"Bangladesh","POP":167.09,"AREA":147.57,"GDP":245.63,"CONT":"Asia","IND_DAY":"1971-03-26"},"RUS":{"COUNTRY":"Russia","POP":146.79,"AREA":17098.25,"GDP":1530.75,"CONT":null,"IND_DAY":"1992-06-12"},"MEX":{"COUNTRY":"Mexico","POP":126.58,"AREA":1964.38,"GDP":1158.23,"CONT":"N.America","IND_DAY":"1810-09-16"},"JPN":{"COUNTRY":"Japan","POP":126.22,"AREA":377.97,"GDP":4872.42,"CONT":"Asia","IND_DAY":null},"DEU":{"COUNTRY":"Germany","POP":83.02,"AREA":357.11,"GDP":3693.2,"CONT":"Europe","IND_DAY":null},"FRA":{"COUNTRY":"France","POP":67.02,"AREA":640.68,"GDP":2582.49,"CONT":"Europe","IND_DAY":"1789-07-14"},"GBR":{"COUNTRY":"UK","POP":66.44,"AREA":242.5,"GDP":2631.23,"CONT":"Europe","IND_DAY":null},"ITA":{"COUNTRY":"Italy","POP":60.36,"AREA":301.34,"GDP":1943.84,"CONT":"Europe","IND_DAY":null},"ARG":{"COUNTRY":"Argentina","POP":44.94,"AREA":2780.4,"GDP":637.49,"CONT":"S.America","IND_DAY":"1816-07-09"},"DZA":{"COUNTRY":"Algeria","POP":43.38,"AREA":2381.74,"GDP":167.56,"CONT":"Africa","IND_DAY":"1962-07-05"},"CAN":{"COUNTRY":"Canada","POP":37.59,"AREA":9984.67,"GDP":1647.12,"CONT":"N.America","IND_DAY":"1867-07-01"},"AUS":{"COUNTRY":"Australia","POP":25.47,"AREA":7692.02,"GDP":1408.68,"CONT":"Oceania","IND_DAY":null},"KAZ":{"COUNTRY":"Kazakhstan","POP":18.53,"AREA":2724.9,"GDP":159.41,"CONT":"Asia","IND_DAY":"1991-12-16"}}
data-index.json
also has one large dictionary, but this time the row labels are the keys, and the inner dictionaries are the values.
There are few more options for orient
. One of them is 'records'
:
>>> df.to_json('data-records.json', orient='records')
This code should yield the file data-records.json
. You can expand the code block below to see the content:
[{"COUNTRY":"China","POP":1398.72,"AREA":9596.96,"GDP":12234.78,"CONT":"Asia","IND_DAY":null},{"COUNTRY":"India","POP":1351.16,"AREA":3287.26,"GDP":2575.67,"CONT":"Asia","IND_DAY":"1947-08-15"},{"COUNTRY":"US","POP":329.74,"AREA":9833.52,"GDP":19485.39,"CONT":"N.America","IND_DAY":"1776-07-04"},{"COUNTRY":"Indonesia","POP":268.07,"AREA":1910.93,"GDP":1015.54,"CONT":"Asia","IND_DAY":"1945-08-17"},{"COUNTRY":"Brazil","POP":210.32,"AREA":8515.77,"GDP":2055.51,"CONT":"S.America","IND_DAY":"1822-09-07"},{"COUNTRY":"Pakistan","POP":205.71,"AREA":881.91,"GDP":302.14,"CONT":"Asia","IND_DAY":"1947-08-14"},{"COUNTRY":"Nigeria","POP":200.96,"AREA":923.77,"GDP":375.77,"CONT":"Africa","IND_DAY":"1960-10-01"},{"COUNTRY":"Bangladesh","POP":167.09,"AREA":147.57,"GDP":245.63,"CONT":"Asia","IND_DAY":"1971-03-26"},{"COUNTRY":"Russia","POP":146.79,"AREA":17098.25,"GDP":1530.75,"CONT":null,"IND_DAY":"1992-06-12"},{"COUNTRY":"Mexico","POP":126.58,"AREA":1964.38,"GDP":1158.23,"CONT":"N.America","IND_DAY":"1810-09-16"},{"COUNTRY":"Japan","POP":126.22,"AREA":377.97,"GDP":4872.42,"CONT":"Asia","IND_DAY":null},{"COUNTRY":"Germany","POP":83.02,"AREA":357.11,"GDP":3693.2,"CONT":"Europe","IND_DAY":null},{"COUNTRY":"France","POP":67.02,"AREA":640.68,"GDP":2582.49,"CONT":"Europe","IND_DAY":"1789-07-14"},{"COUNTRY":"UK","POP":66.44,"AREA":242.5,"GDP":2631.23,"CONT":"Europe","IND_DAY":null},{"COUNTRY":"Italy","POP":60.36,"AREA":301.34,"GDP":1943.84,"CONT":"Europe","IND_DAY":null},{"COUNTRY":"Argentina","POP":44.94,"AREA":2780.4,"GDP":637.49,"CONT":"S.America","IND_DAY":"1816-07-09"},{"COUNTRY":"Algeria","POP":43.38,"AREA":2381.74,"GDP":167.56,"CONT":"Africa","IND_DAY":"1962-07-05"},{"COUNTRY":"Canada","POP":37.59,"AREA":9984.67,"GDP":1647.12,"CONT":"N.America","IND_DAY":"1867-07-01"},{"COUNTRY":"Australia","POP":25.47,"AREA":7692.02,"GDP":1408.68,"CONT":"Oceania","IND_DAY":null},{"COUNTRY":"Kazakhstan","POP":18.53,"AREA":2724.9,"GDP":159.41,"CONT":"Asia","IND_DAY":"1991-12-16"}]
data-records.json
holds a list with one dictionary for each row. The row labels are not written.
You can get another interesting file structure with orient='split'
:
>>> df.to_json('data-split.json', orient='split')
The resulting file is data-split.json
. You can expand the code block below to see how this file should look:
{"columns":["COUNTRY","POP","AREA","GDP","CONT","IND_DAY"],"index":["CHN","IND","USA","IDN","BRA","PAK","NGA","BGD","RUS","MEX","JPN","DEU","FRA","GBR","ITA","ARG","DZA","CAN","AUS","KAZ"],"data":[["China",1398.72,9596.96,12234.78,"Asia",null],["India",1351.16,3287.26,2575.67,"Asia","1947-08-15"],["US",329.74,9833.52,19485.39,"N.America","1776-07-04"],["Indonesia",268.07,1910.93,1015.54,"Asia","1945-08-17"],["Brazil",210.32,8515.77,2055.51,"S.America","1822-09-07"],["Pakistan",205.71,881.91,302.14,"Asia","1947-08-14"],["Nigeria",200.96,923.77,375.77,"Africa","1960-10-01"],["Bangladesh",167.09,147.57,245.63,"Asia","1971-03-26"],["Russia",146.79,17098.25,1530.75,null,"1992-06-12"],["Mexico",126.58,1964.38,1158.23,"N.America","1810-09-16"],["Japan",126.22,377.97,4872.42,"Asia",null],["Germany",83.02,357.11,3693.2,"Europe",null],["France",67.02,640.68,2582.49,"Europe","1789-07-14"],["UK",66.44,242.5,2631.23,"Europe",null],["Italy",60.36,301.34,1943.84,"Europe",null],["Argentina",44.94,2780.4,637.49,"S.America","1816-07-09"],["Algeria",43.38,2381.74,167.56,"Africa","1962-07-05"],["Canada",37.59,9984.67,1647.12,"N.America","1867-07-01"],["Australia",25.47,7692.02,1408.68,"Oceania",null],["Kazakhstan",18.53,2724.9,159.41,"Asia","1991-12-16"]]}
data-split.json
contains one dictionary that holds the following lists:
- The names of the columns
- The labels of the rows
- The inner lists (two-dimensional sequence) that hold data values
If you don’t provide the value for the optional parameter path_or_buf
that defines the file path, then .to_json()
will return a JSON string instead of writing the results to a file. This behavior is consistent with .to_csv()
.
There are other optional parameters you can use. For instance, you can set index=False
to forgo saving row labels. You can manipulate precision with double_precision
, and dates with date_format
and date_unit
. These last two parameters are particularly important when you have time series among your data:
>>> df = pd.DataFrame(data=data).T
>>> df['IND_DAY'] = pd.to_datetime(df['IND_DAY'])
>>> df.dtypes
COUNTRY object
POP object
AREA object
GDP object
CONT object
IND_DAY datetime64[ns]
dtype: object
>>> df.to_json('data-time.json')
In this example, you’ve created the DataFrame
from the dictionary data
and used to_datetime()
to convert the values in the last column to datetime64
. You can expand the code block below to see the resulting file:
{"COUNTRY":{"CHN":"China","IND":"India","USA":"US","IDN":"Indonesia","BRA":"Brazil","PAK":"Pakistan","NGA":"Nigeria","BGD":"Bangladesh","RUS":"Russia","MEX":"Mexico","JPN":"Japan","DEU":"Germany","FRA":"France","GBR":"UK","ITA":"Italy","ARG":"Argentina","DZA":"Algeria","CAN":"Canada","AUS":"Australia","KAZ":"Kazakhstan"},"POP":{"CHN":1398.72,"IND":1351.16,"USA":329.74,"IDN":268.07,"BRA":210.32,"PAK":205.71,"NGA":200.96,"BGD":167.09,"RUS":146.79,"MEX":126.58,"JPN":126.22,"DEU":83.02,"FRA":67.02,"GBR":66.44,"ITA":60.36,"ARG":44.94,"DZA":43.38,"CAN":37.59,"AUS":25.47,"KAZ":18.53},"AREA":{"CHN":9596.96,"IND":3287.26,"USA":9833.52,"IDN":1910.93,"BRA":8515.77,"PAK":881.91,"NGA":923.77,"BGD":147.57,"RUS":17098.25,"MEX":1964.38,"JPN":377.97,"DEU":357.11,"FRA":640.68,"GBR":242.5,"ITA":301.34,"ARG":2780.4,"DZA":2381.74,"CAN":9984.67,"AUS":7692.02,"KAZ":2724.9},"GDP":{"CHN":12234.78,"IND":2575.67,"USA":19485.39,"IDN":1015.54,"BRA":2055.51,"PAK":302.14,"NGA":375.77,"BGD":245.63,"RUS":1530.75,"MEX":1158.23,"JPN":4872.42,"DEU":3693.2,"FRA":2582.49,"GBR":2631.23,"ITA":1943.84,"ARG":637.49,"DZA":167.56,"CAN":1647.12,"AUS":1408.68,"KAZ":159.41},"CONT":{"CHN":"Asia","IND":"Asia","USA":"N.America","IDN":"Asia","BRA":"S.America","PAK":"Asia","NGA":"Africa","BGD":"Asia","RUS":null,"MEX":"N.America","JPN":"Asia","DEU":"Europe","FRA":"Europe","GBR":"Europe","ITA":"Europe","ARG":"S.America","DZA":"Africa","CAN":"N.America","AUS":"Oceania","KAZ":"Asia"},"IND_DAY":{"CHN":null,"IND":-706320000000,"USA":-6106060800000,"IDN":-769219200000,"BRA":-4648924800000,"PAK":-706406400000,"NGA":-291945600000,"BGD":38793600000,"RUS":708307200000,"MEX":-5026838400000,"JPN":null,"DEU":null,"FRA":-5694969600000,"GBR":null,"ITA":null,"ARG":-4843411200000,"DZA":-236476800000,"CAN":-3234729600000,"AUS":null,"KAZ":692841600000}}
In this file, you have large integers instead of dates for the independence days. That’s because the default value of the optional parameter date_format
is 'epoch'
whenever orient
isn’t 'table'
. This default behavior expresses dates as an epoch in milliseconds relative to midnight on January 1, 1970.
However, if you pass date_format='iso'
, then you’ll get the dates in the ISO 8601 format. In addition, date_unit
decides the units of time:
>>> df = pd.DataFrame(data=data).T
>>> df['IND_DAY'] = pd.to_datetime(df['IND_DAY'])
>>> df.to_json('new-data-time.json', date_format='iso', date_unit='s')
This code produces the following JSON file:
{"COUNTRY":{"CHN":"China","IND":"India","USA":"US","IDN":"Indonesia","BRA":"Brazil","PAK":"Pakistan","NGA":"Nigeria","BGD":"Bangladesh","RUS":"Russia","MEX":"Mexico","JPN":"Japan","DEU":"Germany","FRA":"France","GBR":"UK","ITA":"Italy","ARG":"Argentina","DZA":"Algeria","CAN":"Canada","AUS":"Australia","KAZ":"Kazakhstan"},"POP":{"CHN":1398.72,"IND":1351.16,"USA":329.74,"IDN":268.07,"BRA":210.32,"PAK":205.71,"NGA":200.96,"BGD":167.09,"RUS":146.79,"MEX":126.58,"JPN":126.22,"DEU":83.02,"FRA":67.02,"GBR":66.44,"ITA":60.36,"ARG":44.94,"DZA":43.38,"CAN":37.59,"AUS":25.47,"KAZ":18.53},"AREA":{"CHN":9596.96,"IND":3287.26,"USA":9833.52,"IDN":1910.93,"BRA":8515.77,"PAK":881.91,"NGA":923.77,"BGD":147.57,"RUS":17098.25,"MEX":1964.38,"JPN":377.97,"DEU":357.11,"FRA":640.68,"GBR":242.5,"ITA":301.34,"ARG":2780.4,"DZA":2381.74,"CAN":9984.67,"AUS":7692.02,"KAZ":2724.9},"GDP":{"CHN":12234.78,"IND":2575.67,"USA":19485.39,"IDN":1015.54,"BRA":2055.51,"PAK":302.14,"NGA":375.77,"BGD":245.63,"RUS":1530.75,"MEX":1158.23,"JPN":4872.42,"DEU":3693.2,"FRA":2582.49,"GBR":2631.23,"ITA":1943.84,"ARG":637.49,"DZA":167.56,"CAN":1647.12,"AUS":1408.68,"KAZ":159.41},"CONT":{"CHN":"Asia","IND":"Asia","USA":"N.America","IDN":"Asia","BRA":"S.America","PAK":"Asia","NGA":"Africa","BGD":"Asia","RUS":null,"MEX":"N.America","JPN":"Asia","DEU":"Europe","FRA":"Europe","GBR":"Europe","ITA":"Europe","ARG":"S.America","DZA":"Africa","CAN":"N.America","AUS":"Oceania","KAZ":"Asia"},"IND_DAY":{"CHN":null,"IND":"1947-08-15T00:00:00Z","USA":"1776-07-04T00:00:00Z","IDN":"1945-08-17T00:00:00Z","BRA":"1822-09-07T00:00:00Z","PAK":"1947-08-14T00:00:00Z","NGA":"1960-10-01T00:00:00Z","BGD":"1971-03-26T00:00:00Z","RUS":"1992-06-12T00:00:00Z","MEX":"1810-09-16T00:00:00Z","JPN":null,"DEU":null,"FRA":"1789-07-14T00:00:00Z","GBR":null,"ITA":null,"ARG":"1816-07-09T00:00:00Z","DZA":"1962-07-05T00:00:00Z","CAN":"1867-07-01T00:00:00Z","AUS":null,"KAZ":"1991-12-16T00:00:00Z"}}
The dates in the resulting file are in the ISO 8601 format.
You can load the data from a JSON file with read_json()
:
>>> df = pd.read_json('data-index.json', orient='index',
... convert_dates=['IND_DAY'])
The parameter convert_dates
has a similar purpose as parse_dates
when you use it to read CSV files. The optional parameter orient
is very important because it specifies how Pandas understands the structure of the file.
There are other optional parameters you can use as well:
- Set the encoding with
encoding
. - Manipulate dates with
convert_dates
andkeep_default_dates
. - Impact precision with
dtype
andprecise_float
. - Decode numeric data directly to NumPy arrays with
numpy=True
.
Note that you might lose the order of rows and columns when using the JSON format to store your data.
HTML Files
An HTML is a plaintext file that uses hypertext markup language to help browsers render web pages. The extensions for HTML files are .html
and .htm
. You’ll need to install an HTML parser library like lxml or html5lib to be able to work with HTML files:
$pip install lxml html5lib
You can also use Conda to install the same packages:
$ conda install lxml html5lib
Once you have these libraries, you can save the contents of your DataFrame
as an HTML file with .to_html()
:
df = pd.DataFrame(data=data).T
df.to_html('data.html')
This code generates a file data.html
. You can expand the code block below to see how this file should look:
<table border="1" class="dataframe">
<thead>
<tr style="text-align: right;">
<th></th>
<th>COUNTRY</th>
<th>POP</th>
<th>AREA</th>
<th>GDP</th>
<th>CONT</th>
<th>IND_DAY</th>
</tr>
</thead>
<tbody>
<tr>
<th>CHN</th>
<td>China</td>
<td>1398.72</td>
<td>9596.96</td>
<td>12234.8</td>
<td>Asia</td>
<td>NaN</td>
</tr>
<tr>
<th>IND</th>
<td>India</td>
<td>1351.16</td>
<td>3287.26</td>
<td>2575.67</td>
<td>Asia</td>
<td>1947-08-15</td>
</tr>
<tr>
<th>USA</th>
<td>US</td>
<td>329.74</td>
<td>9833.52</td>
<td>19485.4</td>
<td>N.America</td>
<td>1776-07-04</td>
</tr>
<tr>
<th>IDN</th>
<td>Indonesia</td>
<td>268.07</td>
<td>1910.93</td>
<td>1015.54</td>
<td>Asia</td>
<td>1945-08-17</td>
</tr>
<tr>
<th>BRA</th>
<td>Brazil</td>
<td>210.32</td>
<td>8515.77</td>
<td>2055.51</td>
<td>S.America</td>
<td>1822-09-07</td>
</tr>
<tr>
<th>PAK</th>
<td>Pakistan</td>
<td>205.71</td>
<td>881.91</td>
<td>302.14</td>
<td>Asia</td>
<td>1947-08-14</td>
</tr>
<tr>
<th>NGA</th>
<td>Nigeria</td>
<td>200.96</td>
<td>923.77</td>
<td>375.77</td>
<td>Africa</td>
<td>1960-10-01</td>
</tr>
<tr>
<th>BGD</th>
<td>Bangladesh</td>
<td>167.09</td>
<td>147.57</td>
<td>245.63</td>
<td>Asia</td>
<td>1971-03-26</td>
</tr>
<tr>
<th>RUS</th>
<td>Russia</td>
<td>146.79</td>
<td>17098.2</td>
<td>1530.75</td>
<td>NaN</td>
<td>1992-06-12</td>
</tr>
<tr>
<th>MEX</th>
<td>Mexico</td>
<td>126.58</td>
<td>1964.38</td>
<td>1158.23</td>
<td>N.America</td>
<td>1810-09-16</td>
</tr>
<tr>
<th>JPN</th>
<td>Japan</td>
<td>126.22</td>
<td>377.97</td>
<td>4872.42</td>
<td>Asia</td>
<td>NaN</td>
</tr>
<tr>
<th>DEU</th>
<td>Germany</td>
<td>83.02</td>
<td>357.11</td>
<td>3693.2</td>
<td>Europe</td>
<td>NaN</td>
</tr>
<tr>
<th>FRA</th>
<td>France</td>
<td>67.02</td>
<td>640.68</td>
<td>2582.49</td>
<td>Europe</td>
<td>1789-07-14</td>
</tr>
<tr>
<th>GBR</th>
<td>UK</td>
<td>66.44</td>
<td>242.5</td>
<td>2631.23</td>
<td>Europe</td>
<td>NaN</td>
</tr>
<tr>
<th>ITA</th>
<td>Italy</td>
<td>60.36</td>
<td>301.34</td>
<td>1943.84</td>
<td>Europe</td>
<td>NaN</td>
</tr>
<tr>
<th>ARG</th>
<td>Argentina</td>
<td>44.94</td>
<td>2780.4</td>
<td>637.49</td>
<td>S.America</td>
<td>1816-07-09</td>
</tr>
<tr>
<th>DZA</th>
<td>Algeria</td>
<td>43.38</td>
<td>2381.74</td>
<td>167.56</td>
<td>Africa</td>
<td>1962-07-05</td>
</tr>
<tr>
<th>CAN</th>
<td>Canada</td>
<td>37.59</td>
<td>9984.67</td>
<td>1647.12</td>
<td>N.America</td>
<td>1867-07-01</td>
</tr>
<tr>
<th>AUS</th>
<td>Australia</td>
<td>25.47</td>
<td>7692.02</td>
<td>1408.68</td>
<td>Oceania</td>
<td>NaN</td>
</tr>
<tr>
<th>KAZ</th>
<td>Kazakhstan</td>
<td>18.53</td>
<td>2724.9</td>
<td>159.41</td>
<td>Asia</td>
<td>1991-12-16</td>
</tr>
</tbody>
</table>
This file shows the DataFrame
contents nicely. However, notice that you haven’t obtained an entire web page. You’ve just output the data that corresponds to df
in the HTML format.
.to_html()
won’t create a file if you don’t provide the optional parameter buf
, which denotes the buffer to write to. If you leave this parameter out, then your code will return a string as it did with .to_csv()
and .to_json()
.
Here are some other optional parameters:
header
determines whether to save the column names.index
determines whether to save the row labels.classes
assigns cascading style sheet (CSS) classes.render_links
specifies whether to convert URLs to HTML links.table_id
assigns the CSSid
to thetable
tag.escape
decides whether to convert the characters<
,>
, and&
to HTML-safe strings.
You use parameters like these to specify different aspects of the resulting files or strings.
You can create a DataFrame
object from a suitable HTML file using read_html()
, which will return a DataFrame
instance or a list of them:
>>> df = pd.read_html('data.html', index_col=0, parse_dates=['IND_DAY'])
This is very similar to what you did when reading CSV files. You also have parameters that help you work with dates, missing values, precision, encoding, HTML parsers, and more.
Excel Files
You’ve already learned how to read and write Excel files with Pandas. However, there are a few more options worth considering. For one, when you use .to_excel()
, you can specify the name of the target worksheet with the optional parameter sheet_name
:
>>> df = pd.DataFrame(data=data).T
>>> df.to_excel('data.xlsx', sheet_name='COUNTRIES')
Here, you create a file data.xlsx
with a worksheet called COUNTRIES
that stores the data. The string 'data.xlsx'
is the argument for the parameter excel_writer
that defines the name of the Excel file or its path.
The optional parameters startrow
and startcol
both default to 0
and indicate the upper left-most cell where the data should start being written:
>>> df.to_excel('data-shifted.xlsx', sheet_name='COUNTRIES',
... startrow=2, startcol=4)
Here, you specify that the table should start in the third row and the fifth column. You also used zero-based indexing, so the third row is denoted by 2
and the fifth column by 4
.
Now the resulting worksheet looks like this:
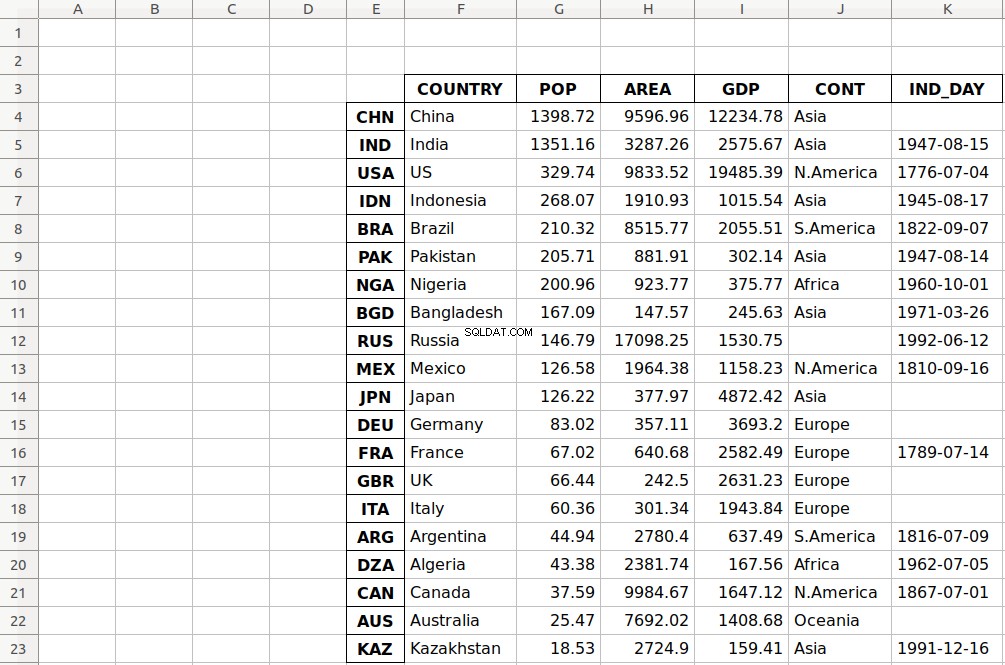
As you can see, the table starts in the third row 2
and the fifth column E
.
.read_excel()
also has the optional parameter sheet_name
that specifies which worksheets to read when loading data. It can take on one of the following values:
- The zero-based index of the worksheet
- The name of the worksheet
- The list of indices or names to read multiple sheets
- The value
None
to read all sheets
Here’s how you would use this parameter in your code:
>>>>>> df = pd.read_excel('data.xlsx', sheet_name=0, index_col=0,
... parse_dates=['IND_DAY'])
>>> df = pd.read_excel('data.xlsx', sheet_name='COUNTRIES', index_col=0,
... parse_dates=['IND_DAY'])
Both statements above create the same DataFrame
because the sheet_name
parameters have the same values. In both cases, sheet_name=0
and sheet_name='COUNTRIES'
refer to the same worksheet. The argument parse_dates=['IND_DAY']
tells Pandas to try to consider the values in this column as dates or times.
There are other optional parameters you can use with .read_excel()
and .to_excel()
to determine the Excel engine, the encoding, the way to handle missing values and infinities, the method for writing column names and row labels, and so on.
SQL Files
Pandas IO tools can also read and write databases. In this next example, you’ll write your data to a database called data.db
. To get started, you’ll need the SQLAlchemy package. To learn more about it, you can read the official ORM tutorial. You’ll also need the database driver. Python has a built-in driver for SQLite.
You can install SQLAlchemy with pip:
$ pip install sqlalchemy
You can also install it with Conda:
$ conda install sqlalchemy
Once you have SQLAlchemy installed, import create_engine()
and create a database engine:
>>> from sqlalchemy import create_engine
>>> engine = create_engine('sqlite:///data.db', echo=False)
Now that you have everything set up, the next step is to create a DataFrame
voorwerp. It’s convenient to specify the data types and apply .to_sql()
.
>>> dtypes = {'POP': 'float64', 'AREA': 'float64', 'GDP': 'float64',
... 'IND_DAY': 'datetime64'}
>>> df = pd.DataFrame(data=data).T.astype(dtype=dtypes)
>>> df.dtypes
COUNTRY object
POP float64
AREA float64
GDP float64
CONT object
IND_DAY datetime64[ns]
dtype: object
.astype()
is a very convenient method you can use to set multiple data types at once.
Once you’ve created your DataFrame
, you can save it to the database with .to_sql()
:
>>> df.to_sql('data.db', con=engine, index_label='ID')
The parameter con
is used to specify the database connection or engine that you want to use. The optional parameter index_label
specifies how to call the database column with the row labels. You’ll often see it take on the value ID
, Id
, or id
.
You should get the database data.db
with a single table that looks like this:
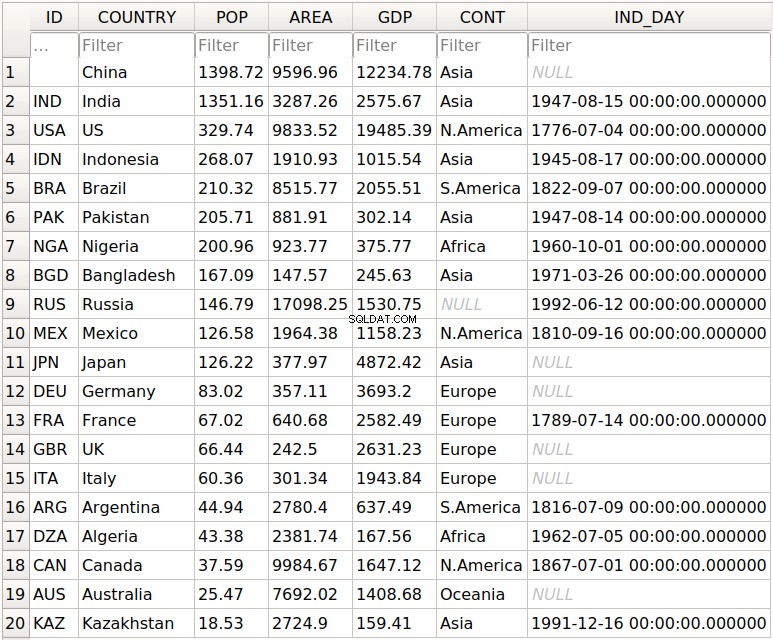
The first column contains the row labels. To omit writing them into the database, pass index=False
to .to_sql()
. The other columns correspond to the columns of the DataFrame
.
There are a few more optional parameters. For example, you can use schema
to specify the database schema and dtype
to determine the types of the database columns. You can also use if_exists
, which says what to do if a database with the same name and path already exists:
if_exists='fail'
raises a ValueError and is the default.if_exists='replace'
drops the table and inserts new values.if_exists='append'
inserts new values into the table.
You can load the data from the database with read_sql()
:
>>> df = pd.read_sql('data.db', con=engine, index_col='ID')
>>> df
COUNTRY POP AREA GDP CONT IND_DAY
ID
CHN China 1398.72 9596.96 12234.78 Asia NaT
IND India 1351.16 3287.26 2575.67 Asia 1947-08-15
USA US 329.74 9833.52 19485.39 N.America 1776-07-04
IDN Indonesia 268.07 1910.93 1015.54 Asia 1945-08-17
BRA Brazil 210.32 8515.77 2055.51 S.America 1822-09-07
PAK Pakistan 205.71 881.91 302.14 Asia 1947-08-14
NGA Nigeria 200.96 923.77 375.77 Africa 1960-10-01
BGD Bangladesh 167.09 147.57 245.63 Asia 1971-03-26
RUS Russia 146.79 17098.25 1530.75 None 1992-06-12
MEX Mexico 126.58 1964.38 1158.23 N.America 1810-09-16
JPN Japan 126.22 377.97 4872.42 Asia NaT
DEU Germany 83.02 357.11 3693.20 Europe NaT
FRA France 67.02 640.68 2582.49 Europe 1789-07-14
GBR UK 66.44 242.50 2631.23 Europe NaT
ITA Italy 60.36 301.34 1943.84 Europe NaT
ARG Argentina 44.94 2780.40 637.49 S.America 1816-07-09
DZA Algeria 43.38 2381.74 167.56 Africa 1962-07-05
CAN Canada 37.59 9984.67 1647.12 N.America 1867-07-01
AUS Australia 25.47 7692.02 1408.68 Oceania NaT
KAZ Kazakhstan 18.53 2724.90 159.41 Asia 1991-12-16
The parameter index_col
specifies the name of the column with the row labels. Note that this inserts an extra row after the header that starts with ID
. You can fix this behavior with the following line of code:
>>> df.index.name = None
>>> df
COUNTRY POP AREA GDP CONT IND_DAY
CHN China 1398.72 9596.96 12234.78 Asia NaT
IND India 1351.16 3287.26 2575.67 Asia 1947-08-15
USA US 329.74 9833.52 19485.39 N.America 1776-07-04
IDN Indonesia 268.07 1910.93 1015.54 Asia 1945-08-17
BRA Brazil 210.32 8515.77 2055.51 S.America 1822-09-07
PAK Pakistan 205.71 881.91 302.14 Asia 1947-08-14
NGA Nigeria 200.96 923.77 375.77 Africa 1960-10-01
BGD Bangladesh 167.09 147.57 245.63 Asia 1971-03-26
RUS Russia 146.79 17098.25 1530.75 None 1992-06-12
MEX Mexico 126.58 1964.38 1158.23 N.America 1810-09-16
JPN Japan 126.22 377.97 4872.42 Asia NaT
DEU Germany 83.02 357.11 3693.20 Europe NaT
FRA France 67.02 640.68 2582.49 Europe 1789-07-14
GBR UK 66.44 242.50 2631.23 Europe NaT
ITA Italy 60.36 301.34 1943.84 Europe NaT
ARG Argentina 44.94 2780.40 637.49 S.America 1816-07-09
DZA Algeria 43.38 2381.74 167.56 Africa 1962-07-05
CAN Canada 37.59 9984.67 1647.12 N.America 1867-07-01
AUS Australia 25.47 7692.02 1408.68 Oceania NaT
KAZ Kazakhstan 18.53 2724.90 159.41 Asia 1991-12-16
Now you have the same DataFrame
object as before.
Note that the continent for Russia is now None
instead of nan
. If you want to fill the missing values with nan
, then you can use .fillna()
:
>>> df.fillna(value=float('nan'), inplace=True)
.fillna()
replaces all missing values with whatever you pass to value
. Here, you passed float('nan')
, which says to fill all missing values with nan
.
Also note that you didn’t have to pass parse_dates=['IND_DAY']
to read_sql()
. That’s because your database was able to detect that the last column contains dates. However, you can pass parse_dates
if you’d like. You’ll get the same results.
There are other functions that you can use to read databases, like read_sql_table()
and read_sql_query()
. Feel free to try them out!
Pickle Files
Pickling is the act of converting Python objects into byte streams. Unpickling is the inverse process. Python pickle files are the binary files that keep the data and hierarchy of Python objects. They usually have the extension .pickle
or .pkl
.
You can save your DataFrame
in a pickle file with .to_pickle()
:
>>> dtypes = {'POP': 'float64', 'AREA': 'float64', 'GDP': 'float64',
... 'IND_DAY': 'datetime64'}
>>> df = pd.DataFrame(data=data).T.astype(dtype=dtypes)
>>> df.to_pickle('data.pickle')
Like you did with databases, it can be convenient first to specify the data types. Then, you create a file data.pickle
to contain your data. You could also pass an integer value to the optional parameter protocol
, which specifies the protocol of the pickler.
You can get the data from a pickle file with read_pickle()
:
>>> df = pd.read_pickle('data.pickle')
>>> df
COUNTRY POP AREA GDP CONT IND_DAY
CHN China 1398.72 9596.96 12234.78 Asia NaT
IND India 1351.16 3287.26 2575.67 Asia 1947-08-15
USA US 329.74 9833.52 19485.39 N.America 1776-07-04
IDN Indonesia 268.07 1910.93 1015.54 Asia 1945-08-17
BRA Brazil 210.32 8515.77 2055.51 S.America 1822-09-07
PAK Pakistan 205.71 881.91 302.14 Asia 1947-08-14
NGA Nigeria 200.96 923.77 375.77 Africa 1960-10-01
BGD Bangladesh 167.09 147.57 245.63 Asia 1971-03-26
RUS Russia 146.79 17098.25 1530.75 NaN 1992-06-12
MEX Mexico 126.58 1964.38 1158.23 N.America 1810-09-16
JPN Japan 126.22 377.97 4872.42 Asia NaT
DEU Germany 83.02 357.11 3693.20 Europe NaT
FRA France 67.02 640.68 2582.49 Europe 1789-07-14
GBR UK 66.44 242.50 2631.23 Europe NaT
ITA Italy 60.36 301.34 1943.84 Europe NaT
ARG Argentina 44.94 2780.40 637.49 S.America 1816-07-09
DZA Algeria 43.38 2381.74 167.56 Africa 1962-07-05
CAN Canada 37.59 9984.67 1647.12 N.America 1867-07-01
AUS Australia 25.47 7692.02 1408.68 Oceania NaT
KAZ Kazakhstan 18.53 2724.90 159.41 Asia 1991-12-16
read_pickle()
returns the DataFrame
with the stored data. You can also check the data types:
>>> df.dtypes
COUNTRY object
POP float64
AREA float64
GDP float64
CONT object
IND_DAY datetime64[ns]
dtype: object
These are the same ones that you specified before using .to_pickle()
.
As a word of caution, you should always beware of loading pickles from untrusted sources. This can be dangerous! When you unpickle an untrustworthy file, it could execute arbitrary code on your machine, gain remote access to your computer, or otherwise exploit your device in other ways.
Working With Big Data
If your files are too large for saving or processing, then there are several approaches you can take to reduce the required disk space:
- Compress your files
- Choose only the columns you want
- Omit the rows you don’t need
- Force the use of less precise data types
- Split the data into chunks
You’ll take a look at each of these techniques in turn.
Compress and Decompress Files
You can create an archive file like you would a regular one, with the addition of a suffix that corresponds to the desired compression type:
'.gz'
'.bz2'
'.zip'
'.xz'
Pandas can deduce the compression type by itself:
>>>>>> df = pd.DataFrame(data=data).T
>>> df.to_csv('data.csv.zip')
Here, you create a compressed .csv
file as an archive. The size of the regular .csv
file is 1048 bytes, while the compressed file only has 766 bytes.
You can open this compressed file as usual with the Pandas read_csv()
functie:
>>> df = pd.read_csv('data.csv.zip', index_col=0,
... parse_dates=['IND_DAY'])
>>> df
COUNTRY POP AREA GDP CONT IND_DAY
CHN China 1398.72 9596.96 12234.78 Asia NaT
IND India 1351.16 3287.26 2575.67 Asia 1947-08-15
USA US 329.74 9833.52 19485.39 N.America 1776-07-04
IDN Indonesia 268.07 1910.93 1015.54 Asia 1945-08-17
BRA Brazil 210.32 8515.77 2055.51 S.America 1822-09-07
PAK Pakistan 205.71 881.91 302.14 Asia 1947-08-14
NGA Nigeria 200.96 923.77 375.77 Africa 1960-10-01
BGD Bangladesh 167.09 147.57 245.63 Asia 1971-03-26
RUS Russia 146.79 17098.25 1530.75 NaN 1992-06-12
MEX Mexico 126.58 1964.38 1158.23 N.America 1810-09-16
JPN Japan 126.22 377.97 4872.42 Asia NaT
DEU Germany 83.02 357.11 3693.20 Europe NaT
FRA France 67.02 640.68 2582.49 Europe 1789-07-14
GBR UK 66.44 242.50 2631.23 Europe NaT
ITA Italy 60.36 301.34 1943.84 Europe NaT
ARG Argentina 44.94 2780.40 637.49 S.America 1816-07-09
DZA Algeria 43.38 2381.74 167.56 Africa 1962-07-05
CAN Canada 37.59 9984.67 1647.12 N.America 1867-07-01
AUS Australia 25.47 7692.02 1408.68 Oceania NaT
KAZ Kazakhstan 18.53 2724.90 159.41 Asia 1991-12-16
read_csv()
decompresses the file before reading it into a DataFrame
.
You can specify the type of compression with the optional parameter compression
, which can take on any of the following values:
'infer'
'gzip'
'bz2'
'zip'
'xz'
None
The default value compression='infer'
indicates that Pandas should deduce the compression type from the file extension.
Here’s how you would compress a pickle file:
>>>>>> df = pd.DataFrame(data=data).T
>>> df.to_pickle('data.pickle.compress', compression='gzip')
You should get the file data.pickle.compress
that you can later decompress and read:
>>> df = pd.read_pickle('data.pickle.compress', compression='gzip')
df
again corresponds to the DataFrame
with the same data as before.
You can give the other compression methods a try, as well. If you’re using pickle files, then keep in mind that the .zip
format supports reading only.
Choose Columns
The Pandas read_csv()
and read_excel()
functions have the optional parameter usecols
that you can use to specify the columns you want to load from the file. You can pass the list of column names as the corresponding argument:
>>> df = pd.read_csv('data.csv', usecols=['COUNTRY', 'AREA'])
>>> df
COUNTRY AREA
0 China 9596.96
1 India 3287.26
2 US 9833.52
3 Indonesia 1910.93
4 Brazil 8515.77
5 Pakistan 881.91
6 Nigeria 923.77
7 Bangladesh 147.57
8 Russia 17098.25
9 Mexico 1964.38
10 Japan 377.97
11 Germany 357.11
12 France 640.68
13 UK 242.50
14 Italy 301.34
15 Argentina 2780.40
16 Algeria 2381.74
17 Canada 9984.67
18 Australia 7692.02
19 Kazakhstan 2724.90
Now you have a DataFrame
that contains less data than before. Here, there are only the names of the countries and their areas.
Instead of the column names, you can also pass their indices:
>>>>>> df = pd.read_csv('data.csv',index_col=0, usecols=[0, 1, 3])
>>> df
COUNTRY AREA
CHN China 9596.96
IND India 3287.26
USA US 9833.52
IDN Indonesia 1910.93
BRA Brazil 8515.77
PAK Pakistan 881.91
NGA Nigeria 923.77
BGD Bangladesh 147.57
RUS Russia 17098.25
MEX Mexico 1964.38
JPN Japan 377.97
DEU Germany 357.11
FRA France 640.68
GBR UK 242.50
ITA Italy 301.34
ARG Argentina 2780.40
DZA Algeria 2381.74
CAN Canada 9984.67
AUS Australia 7692.02
KAZ Kazakhstan 2724.90
Expand the code block below to compare these results with the file 'data.csv'
:
,COUNTRY,POP,AREA,GDP,CONT,IND_DAY
CHN,China,1398.72,9596.96,12234.78,Asia,
IND,India,1351.16,3287.26,2575.67,Asia,1947-08-15
USA,US,329.74,9833.52,19485.39,N.America,1776-07-04
IDN,Indonesia,268.07,1910.93,1015.54,Asia,1945-08-17
BRA,Brazil,210.32,8515.77,2055.51,S.America,1822-09-07
PAK,Pakistan,205.71,881.91,302.14,Asia,1947-08-14
NGA,Nigeria,200.96,923.77,375.77,Africa,1960-10-01
BGD,Bangladesh,167.09,147.57,245.63,Asia,1971-03-26
RUS,Russia,146.79,17098.25,1530.75,,1992-06-12
MEX,Mexico,126.58,1964.38,1158.23,N.America,1810-09-16
JPN,Japan,126.22,377.97,4872.42,Asia,
DEU,Germany,83.02,357.11,3693.2,Europe,
FRA,France,67.02,640.68,2582.49,Europe,1789-07-14
GBR,UK,66.44,242.5,2631.23,Europe,
ITA,Italy,60.36,301.34,1943.84,Europe,
ARG,Argentina,44.94,2780.4,637.49,S.America,1816-07-09
DZA,Algeria,43.38,2381.74,167.56,Africa,1962-07-05
CAN,Canada,37.59,9984.67,1647.12,N.America,1867-07-01
AUS,Australia,25.47,7692.02,1408.68,Oceania,
KAZ,Kazakhstan,18.53,2724.9,159.41,Asia,1991-12-16
You can see the following columns:
- The column at index
0
contains the row labels. - The column at index
1
contains the country names. - The column at index
3
contains the areas.
Simlarly, read_sql()
has the optional parameter columns
that takes a list of column names to read:
>>> df = pd.read_sql('data.db', con=engine, index_col='ID',
... columns=['COUNTRY', 'AREA'])
>>> df.index.name = None
>>> df
COUNTRY AREA
CHN China 9596.96
IND India 3287.26
USA US 9833.52
IDN Indonesia 1910.93
BRA Brazil 8515.77
PAK Pakistan 881.91
NGA Nigeria 923.77
BGD Bangladesh 147.57
RUS Russia 17098.25
MEX Mexico 1964.38
JPN Japan 377.97
DEU Germany 357.11
FRA France 640.68
GBR UK 242.50
ITA Italy 301.34
ARG Argentina 2780.40
DZA Algeria 2381.74
CAN Canada 9984.67
AUS Australia 7692.02
KAZ Kazakhstan 2724.90
Again, the DataFrame
only contains the columns with the names of the countries and areas. If columns
is None
or omitted, then all of the columns will be read, as you saw before. The default behavior is columns=None
.
Omit Rows
When you test an algorithm for data processing or machine learning, you often don’t need the entire dataset. It’s convenient to load only a subset of the data to speed up the process. The Pandas read_csv()
and read_excel()
functions have some optional parameters that allow you to select which rows you want to load:
skiprows
: either the number of rows to skip at the beginning of the file if it’s an integer, or the zero-based indices of the rows to skip if it’s a list-like objectskipfooter
: the number of rows to skip at the end of the filenrows
: the number of rows to read
Here’s how you would skip rows with odd zero-based indices, keeping the even ones:
>>>>>> df = pd.read_csv('data.csv', index_col=0, skiprows=range(1, 20, 2))
>>> df
COUNTRY POP AREA GDP CONT IND_DAY
IND India 1351.16 3287.26 2575.67 Asia 1947-08-15
IDN Indonesia 268.07 1910.93 1015.54 Asia 1945-08-17
PAK Pakistan 205.71 881.91 302.14 Asia 1947-08-14
BGD Bangladesh 167.09 147.57 245.63 Asia 1971-03-26
MEX Mexico 126.58 1964.38 1158.23 N.America 1810-09-16
DEU Germany 83.02 357.11 3693.20 Europe NaN
GBR UK 66.44 242.50 2631.23 Europe NaN
ARG Argentina 44.94 2780.40 637.49 S.America 1816-07-09
CAN Canada 37.59 9984.67 1647.12 N.America 1867-07-01
KAZ Kazakhstan 18.53 2724.90 159.41 Asia 1991-12-16
In this example, skiprows
is range(1, 20, 2)
and corresponds to the values 1
, 3
, …, 19
. The instances of the Python built-in class range
behave like sequences. The first row of the file data.csv
is the header row. It has the index 0
, so Pandas loads it in. The second row with index 1
corresponds to the label CHN
, and Pandas skips it. The third row with the index 2
and label IND
is loaded, and so on.
If you want to choose rows randomly, then skiprows
can be a list or NumPy array with pseudo-random numbers, obtained either with pure Python or with NumPy.
Force Less Precise Data Types
If you’re okay with less precise data types, then you can potentially save a significant amount of memory! First, get the data types with .dtypes
again:
>>> df = pd.read_csv('data.csv', index_col=0, parse_dates=['IND_DAY'])
>>> df.dtypes
COUNTRY object
POP float64
AREA float64
GDP float64
CONT object
IND_DAY datetime64[ns]
dtype: object
The columns with the floating-point numbers are 64-bit floats. Each number of this type float64
consumes 64 bits or 8 bytes. Each column has 20 numbers and requires 160 bytes. You can verify this with .memory_usage()
:
>>> df.memory_usage()
Index 160
COUNTRY 160
POP 160
AREA 160
GDP 160
CONT 160
IND_DAY 160
dtype: int64
.memory_usage()
returns an instance of Series
with the memory usage of each column in bytes. You can conveniently combine it with .loc[]
and .sum()
to get the memory for a group of columns:
>>> df.loc[:, ['POP', 'AREA', 'GDP']].memory_usage(index=False).sum()
480
This example shows how you can combine the numeric columns 'POP'
, 'AREA'
, and 'GDP'
to get their total memory requirement. The argument index=False
excludes data for row labels from the resulting Series
voorwerp. For these three columns, you’ll need 480 bytes.
You can also extract the data values in the form of a NumPy array with .to_numpy()
or .values
. Then, use the .nbytes
attribute to get the total bytes consumed by the items of the array:
>>> df.loc[:, ['POP', 'AREA', 'GDP']].to_numpy().nbytes
480
The result is the same 480 bytes. So, how do you save memory?
In this case, you can specify that your numeric columns 'POP'
, 'AREA'
, and 'GDP'
should have the type float32
. Use the optional parameter dtype
to do this:
>>> dtypes = {'POP': 'float32', 'AREA': 'float32', 'GDP': 'float32'}
>>> df = pd.read_csv('data.csv', index_col=0, dtype=dtypes,
... parse_dates=['IND_DAY'])
The dictionary dtypes
specifies the desired data types for each column. It’s passed to the Pandas read_csv()
function as the argument that corresponds to the parameter dtype
.
Now you can verify that each numeric column needs 80 bytes, or 4 bytes per item:
>>>>>> df.dtypes
COUNTRY object
POP float32
AREA float32
GDP float32
CONT object
IND_DAY datetime64[ns]
dtype: object
>>> df.memory_usage()
Index 160
COUNTRY 160
POP 80
AREA 80
GDP 80
CONT 160
IND_DAY 160
dtype: int64
>>> df.loc[:, ['POP', 'AREA', 'GDP']].memory_usage(index=False).sum()
240
>>> df.loc[:, ['POP', 'AREA', 'GDP']].to_numpy().nbytes
240
Each value is a floating-point number of 32 bits or 4 bytes. The three numeric columns contain 20 items each. In total, you’ll need 240 bytes of memory when you work with the type float32
. This is half the size of the 480 bytes you’d need to work with float64
.
In addition to saving memory, you can significantly reduce the time required to process data by using float32
instead of float64
in some cases.
Use Chunks to Iterate Through Files
Another way to deal with very large datasets is to split the data into smaller chunks and process one chunk at a time. If you use read_csv()
, read_json()
or read_sql()
, then you can specify the optional parameter chunksize
:
>>> data_chunk = pd.read_csv('data.csv', index_col=0, chunksize=8)
>>> type(data_chunk)
<class 'pandas.io.parsers.TextFileReader'>
>>> hasattr(data_chunk, '__iter__')
True
>>> hasattr(data_chunk, '__next__')
True
chunksize
defaults to None
and can take on an integer value that indicates the number of items in a single chunk. When chunksize
is an integer, read_csv()
returns an iterable that you can use in a for
loop to get and process only a fragment of the dataset in each iteration:
>>> for df_chunk in pd.read_csv('data.csv', index_col=0, chunksize=8):
... print(df_chunk, end='\n\n')
... print('memory:', df_chunk.memory_usage().sum(), 'bytes',
... end='\n\n\n')
...
COUNTRY POP AREA GDP CONT IND_DAY
CHN China 1398.72 9596.96 12234.78 Asia NaN
IND India 1351.16 3287.26 2575.67 Asia 1947-08-15
USA US 329.74 9833.52 19485.39 N.America 1776-07-04
IDN Indonesia 268.07 1910.93 1015.54 Asia 1945-08-17
BRA Brazil 210.32 8515.77 2055.51 S.America 1822-09-07
PAK Pakistan 205.71 881.91 302.14 Asia 1947-08-14
NGA Nigeria 200.96 923.77 375.77 Africa 1960-10-01
BGD Bangladesh 167.09 147.57 245.63 Asia 1971-03-26
memory: 448 bytes
COUNTRY POP AREA GDP CONT IND_DAY
RUS Russia 146.79 17098.25 1530.75 NaN 1992-06-12
MEX Mexico 126.58 1964.38 1158.23 N.America 1810-09-16
JPN Japan 126.22 377.97 4872.42 Asia NaN
DEU Germany 83.02 357.11 3693.20 Europe NaN
FRA France 67.02 640.68 2582.49 Europe 1789-07-14
GBR UK 66.44 242.50 2631.23 Europe NaN
ITA Italy 60.36 301.34 1943.84 Europe NaN
ARG Argentina 44.94 2780.40 637.49 S.America 1816-07-09
memory: 448 bytes
COUNTRY POP AREA GDP CONT IND_DAY
DZA Algeria 43.38 2381.74 167.56 Africa 1962-07-05
CAN Canada 37.59 9984.67 1647.12 N.America 1867-07-01
AUS Australia 25.47 7692.02 1408.68 Oceania NaN
KAZ Kazakhstan 18.53 2724.90 159.41 Asia 1991-12-16
memory: 224 bytes
In this example, the chunksize
is 8
. The first iteration of the for
loop returns a DataFrame
with the first eight rows of the dataset only. The second iteration returns another DataFrame
with the next eight rows. The third and last iteration returns the remaining four rows.
Opmerking: You can also pass iterator=True
to force the Pandas read_csv()
function to return an iterator object instead of a DataFrame
voorwerp.
In each iteration, you get and process the DataFrame
with the number of rows equal to chunksize
. It’s possible to have fewer rows than the value of chunksize
in the last iteration. You can use this functionality to control the amount of memory required to process data and keep that amount reasonably small.
Conclusie
You now know how to save the data and labels from Pandas DataFrame
objects to different kinds of files. You also know how to load your data from files and create DataFrame
objects.
You’ve used the Pandas read_csv()
and .to_csv()
methods to read and write CSV files. You also used similar methods to read and write Excel, JSON, HTML, SQL, and pickle files. These functions are very convenient and widely used. They allow you to save or load your data in a single function or method call.
You’ve also learned how to save time, memory, and disk space when working with large data files:
- Compress or decompress files
- Choose the rows and columns you want to load
- Use less precise data types
- Split data into chunks and process them one by one
You’ve mastered a significant step in the machine learning and data science process! If you have any questions or comments, then please put them in the comments section below.